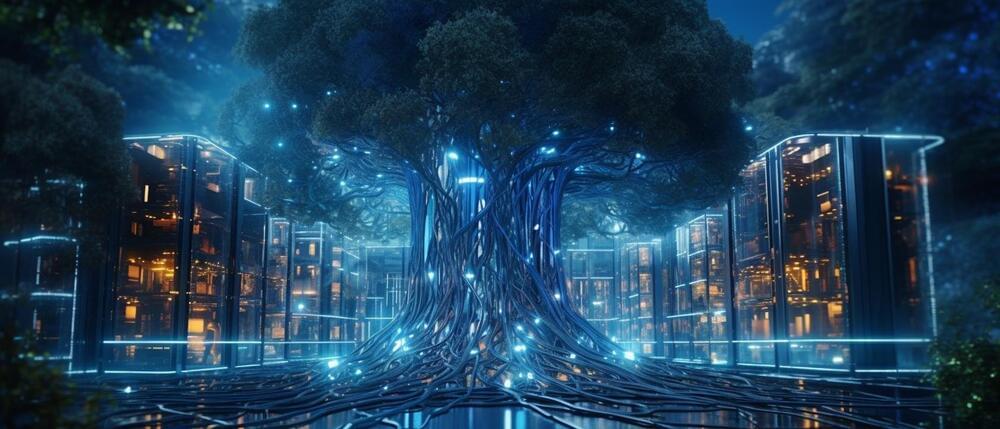
Update: The image for the ChatGPT 3.5 and vicuna-13B comparison has been updated for readability.
With the launch of Large Language Models (LLMs) for Generative Artificial Intelligence (GenAI), the world has become both enamored and concerned with the potential for AI. The ability to hold a conversation, pass a test, develop a research paper, or write software code are tremendous feats of AI, but they are only the beginning to what GenAI will be able to accomplish over the next few years. All this innovative capability comes at a high cost in terms of processing performance and power consumption. So, while the potential for AI may be limitless, physics and costs may ultimately be the boundaries.
Tirias Research forecasts that on the current course, generative AI data center server infrastructure plus operating costs will exceed $76 billion by 2028, with growth challenging the business models and profitability of emergent services such as search, content creation, and business automation incorporating GenAI. For perspective, this cost is more than twice the estimated annual operating cost of Amazon’s cloud service AWS, which today holds one third of the cloud infrastructure services market according to Tirias Research estimates. This forecast incorporates an aggressive 4X improvement in hardware compute performance, but this gain is overrun by a 50X increase in processing workloads, even with a rapid rate of innovation around inference algorithms and their efficiency. Neural Networks (NNs) designed to run at scale will be even more highly optimized and will continue to improve over time, which will increase each server’s capacity. However, this improvement is countered by increasing usage, more demanding use cases, and more sophisticated models with orders of magnitude more parameters. The cost and scale of GenAI will demand innovation in optimizing NNs and is likely to push the computational load out from data centers to client devices like PCs and smartphones.