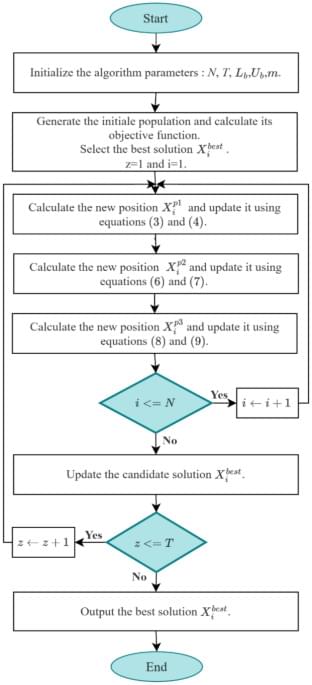
The Driving Training Based Optimization (DTBO) algorithm, proposed by Mohammad Dehghani, is one of the novel metaheuristic algorithms which appeared in 202280. This algorithm is founded on the principle of learning to drive, which unfolds in three phases: selecting an instructor from the learners, receiving instructions from the instructor on driving techniques, and practicing newly learned techniques from the learner to enhance one’s driving abilities81,82. In this work, DTBO algorithm is used, due to its effectiveness, which was confirmed by a comparative study83 with other algorithms, including particle swarm optimization84, Gravitational Search Algorithm (GSA)85, teaching learning-based optimization, Gray Wolf Optimization (GWO)86, Whale Optimization Algorithm (WOA)87, and Reptile Search Algorithm (RSA)88. The comparative study has been done using various kinds of benchmark functions, such as constrained, nonlinear and non-convex functions.
Lyapunov-based Model Predictive Control (LMPC) is a control approach integrating Lyapunov function as constraint in the optimization problem of MPC89,90. This technique characterizes the region of the closed-loop stability, which makes it possible to define the operating conditions that maintain the system stability91,92. Since its appearance, the LMPC method has been utilized extensively for controlling a various nonlinear systems, such as robotic systems93, electrical systems94, chemical processes95, and wind power generation systems90. In contrast to the LMPC, both the regular MPC and the NMPC lack explicit stability restrictions and can’t combine stability guarantees with interpretability, even with their increased flexibility.
The proposed method, named Lyapunov-based neural network model predictive control using metaheuristic optimization approach (LNNMPC-MOA), includes Lyapunov-based constraint in the optimization problem of the neural network model predictive control (NNMPC), which is solved by the DTBO algorithm. The suggested controller consists of two parts: the first is responsible for calculating predictions using a neural network model of the feedforward type, and the second is responsible to resolve the constrained nonlinear optimization problem using the DTBO algorithm. This technique is suggested to solve the nonlinear and non-convex optimization problem of the conventional NMPC, ensure on-line optimization in reasonable time thanks to their easy implementation and guaranty the stability using the Lyapunov function-based constraint. The efficiency of the proposed controller regarding to the accuracy, quickness and robustness is assessed by taking into account the speed control of a three-phase induction motor, and its stability is mathematically ensured using the Lyapunov function-based constraint. The acquired results are compared to those of NNMPC based on DTBO algorithm (NNMPC-DTBO), NNMPC using PSO algorithm (NNMPC-PSO), Fuzzy Logic controller optimized by TLBO (FLC-TLBO) and optimized PID controller using PSO algorithm (PID-PSO)95.