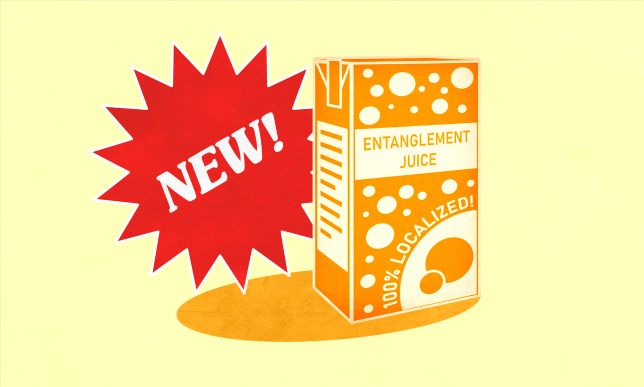
“Conditional witnessing” technique makes many-body entangled states easier to measure.
Quantum error correction – a crucial ingredient in bringing quantum computers into the mainstream – relies on sharing entanglement between many particles at once. Thanks to researchers in the UK, Spain and Germany, measuring those entangled states just got a lot easier. The new measurement procedure, which the researchers term “conditional witnessing”, is more robust to noise than previous techniques and minimizes the number of measurements required, making it a valuable method for testing imperfect real-life quantum systems.
Quantum computers run their algorithms on quantum bits, or qubits. These physical two-level quantum systems play an analogous role to classical bits, except that instead of being restricted to just “0” or “1” states, a single qubit can be in any combination of the two. This extra information capacity, combined with the ability to manipulate quantum entanglement between qubits (thus allowing multiple calculations to be performed simultaneously), is a key advantage of quantum computers.
The problem with qubits
However, qubits are fragile. Virtually any interaction with their environment can cause them to collapse like a house of cards and lose their quantum correlations – a process called decoherence. If this happens before an algorithm finishes running, the result is a mess, not an answer. (You would not get much work done on a laptop that had to restart every second.) In general, the more qubits a quantum computer has, the harder they are to keep quantum; even today’s most advanced quantum processors still have fewer than 100 physical qubits.